by Kausutua Tjikundi
Continuous prevalence and re-emergence of infectious diseases in low-and middle-income countries, especially in Sub-Saharan Africa, necessitate accurate mining and analysis of face-to-face contact patterns between individuals. Most importantly, contact patterns among individuals are essential in identifying the potential transmission routes of infectious diseases, and particularly of respiratory pathogens. Recent technological advancements, such as wearable devices, paved the way to measure and quantify real-world person-to-person interactions, with high resolution in space and time. This allows one to best inform epidemic models, by estimating epidemic risk and to provide insights into the design of intervention and mitigation strategies for social protection. Traditional methods for studying face-to-face interactions (relevant to the transmission of infectious diseases), such as self-reported surveys and contact diaries, often suffer from inaccuracies due to recall bias, underreporting of the frequency of contacts, and overestimation of contact duration. However, wearable sensors are capable of overcoming these limitations by objectively quantifying human interactions. This blog shares some insights on the importance and potential impact of measuring face-to-face interactions using wearable sensors in Sub-Saharan Africa for epidemic spreading control.
A paradigm shift in contact data collection
Computational social sciences paved the way for the use of digital traces to study, monitor, measure and model social systems. Wearable sensors proved to be a powerful tool for collecting detailed data on human proximity and interactions. In particular, in this blog, we focus on the wearable technology developed by the SocioPatterns collaboration (https://www.sociopatterns.org). These devices are unobtrusive lightweight badges worn on the chest and capable of capturing time-resolved data at high spatial and temporal resolutions. They provide high-resolution data on proximity, capturing detailed interaction patterns that were previously hard to quantify. By detecting when individuals engage in a face-to-face contact, these wearable sensors provide an accurate representation of social networks and contact patterns within a population. The sensors make use of low-power radio frequencies, such as RFID technology or other wireless communication methods to detect face-to-face interactions with high temporal (up to 10 seconds) and spatial (within 1-2 meters) resolutions. This technology exploits the principle that the human body acts as a shield for the radio frequencies used, allowing for a precise detection of face-to-face interactions, as opposed to simply being in close proximity. This granular data captures not only the frequency but also the duration and exact timestamps of contacts for a given social setting. This technology addresses and overcomes many limitations associated with traditional data collection methods and holds great potential for application in diverse environments, particularly low-resource settings commonly found in Sub-Saharan Africa.
Burden of Infectious Diseases in Sub-Saharan Africa
Sub-Saharan Africa faces several public health challenges due to its high burden and the emergence of infectious diseases such as tuberculosis, influenza, meningitis, Ebola, respiratory syncytial virus (RSV), pneumonia, and measles. These infectious diseases remain prevalent and continue to strain and disrupt public health in this region. The recent COVID-19 pandemic has added to this burden by further exposing vulnerabilities in public health systems and highlighting the urgent need for sophisticated and robust infectious disease control mechanisms. The dynamics of disease transmission in the region are shaped by distinct social, economic, and environmental factors. Understanding how individuals interact (in close proximity) in daily life is fundamental to predicting how diseases spread within the human population.
Overcoming the limitations of traditional data collection
As mentioned already, traditional methods of gathering social contact data, such as self-reported contact diaries and surveys, often suffer from recall bias and inaccuracies. These methods introduce further difficulties (related to completing surveys) for children and participants who can not read and write. However, these problems do not influence the sensor-based data collection. Individuals may also forget short encounters or fail to report all interactions, leading to incomplete or skewed data. In the context of infectious diseases, where even fleeting contacts can contribute to transmission, these gaps can hinder the effectiveness of epidemiological models and public health interventions. By collecting high-resolution, time-resolved data on human interactions, wearable sensors overcome the limitations of these methods. This approach provides an objective and precise method for capturing real-world social interactions that are relevant and important for modeling the spread of infectious diseases. The access to time-resolved contact data enables the description of potential infection routes across the human population. This, consequently, allows better informed decision-making. The figure below is an example of the importance of having detailed data on face-to-face interactions. The red colour indicates an infectious individual. The first three plots indicate the contacts at three different time-stamps, while the last one is an aggregated picture of the contacts over time.
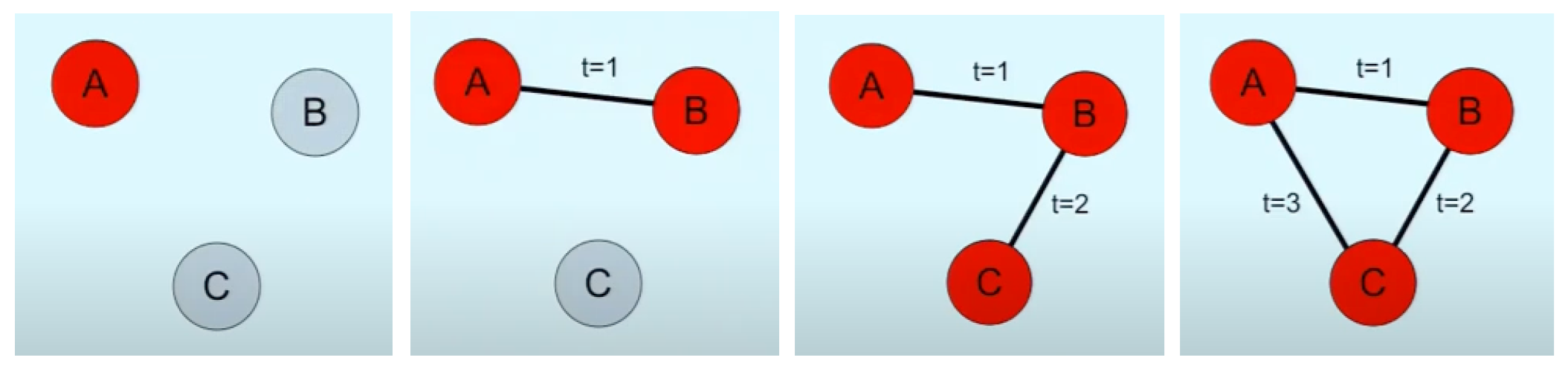
This clearly shows that the fastest path (for instance between A and C) is not the shortest path when dealing with temporal networks. If we had a static network where we did not have time stamps for each contact event then we would assume that the propagation of the infection followed the shortest path, and that is not always true when dealing with temporal networks. Therefore, with time resolution, there is a benefit of seeing how the network is evolving with time, hence it is important to mine face-to-face interaction data with high temporal and spatial resolution.
Addressing data gaps in low-resource settings
One of the key limitations is the scarcity of high-quality data on social contact patterns in low-resource settings like Sub-Saharan Africa. Most studies on social contact patterns relevant to the transmission of infectious diseases focused on developed/ high-income countries, where social structures, healthcare infrastructure, social behaviours, population demographics, and disease transmission patterns differ significantly from those in Africa. In developed countries, data of this nature have been collected and analysed in different settings such as schools, workplaces, hospital wards, museums, households, and conferences. Even if some work has been done on a small scale in countries such as Kenya, Malawi, and South Africa, the contact patterns among individuals remain largely unexplored for most parts of Sub-Saharan Africa. Given that we live in an interconnected world, this lack of information can have major consequences also for the global healthcare system. The COVID-19 pandemic reminded us once again that infectious diseases can reach any corner of the world in a couple of hours, therefore, the precise characterization of contacts in all parts of the world is necessary for effective outbreak mitigation and global pandemic preparedness. Therefore, one of the most important contributions would be to extend the efforts in quantifying face-to-face interactions to other parts of the Sub-Saharan region to bridge the knowledge and data gap. Sub-Saharan Africa presents unique characteristics in every aspect of life, culture and tradition, socio-economic status and other demographic characteristics, for instance in this region household structure differs from other parts of the world and also within the region. A relevant question would be to get a nuanced understanding of how these unique demographics and socioeconomic characteristics shape face-to-face interactions that are relevant to infectious diseases across different settings in the Sub-Saharan African region. The real-world data collected from Sub-Saharan Africa can be integrated into infectious disease models to improve the accuracy of their predictions and develop more effective strategies for controlling disease outbreaks.
Conclusion
Mining face-to-face interactions using wearable sensors has the potential to improve infectious disease control in Sub-Saharan Africa. Detailed, accurate, and time-resolved data on social interactions provided by these technologies can allow researchers and public health officials to gain a better understanding of the dynamics of disease transmission. This approach allows for more targeted, effective, and context-specific interventions, which are important for preventing the spread of infections in regions with limited and stretched healthcare resources. Therefore, investing in close-proximity wearable sensor technology and expanding research efforts to collect and analyze face-to-face interaction data are necessary steps toward strengthening disease surveillance and preparedness in the Sub-Saharan African region. Bridging the current data gaps and understanding how the unique socio-economic and demographic characteristics of the region drive contact patterns can help develop more accurate predictions and effective strategies to combat infectious diseases. However, it is important to acknowledge the limitations of wearable sensors, as they capture data only from individuals who are wearing the devices.
Additional Resources
For more information, visit Sociopatterns Publications.
References
Barrat, A., Cattuto, C., Tozzi, A. E., Vanhems, P., & Voirin, N. (2014). Measuring contact patterns with wearable sensors: Methods, data characteristics and applications to data-driven simulations of infectious diseases. Clinical Microbiology and Infection, 20(1), 10–16.
Cattuto, C., Van den Broeck, W., Barrat, A., Colizza, V., Pinton, J.-F., & Vespignani, A. (2010). Dynamics of person-to-person interactions from distributed rfid sensor networks. PloS one, 5(7), e11596.
Dall’Amico, L., Kleynhans, J., Gauvin, L., Tizzoni, M., Ozella, L., Makhasi, M., Wolter, N., Language, B., Wagner, R. G., Cohen, C., et al. (2024). Estimating household contact matrices structure from easily collectable metadata. Plos one, 19(3), e0296810.
Huang, Y., Cai, X., Zhang, B., Zhu, G., Liu, T., Guo, P., Xiao, J., Li, X., Zeng, W., Hu, J., et al. (2020). Spatiotemporal heterogeneity of social contact patterns related to infectious diseases in the guangdong province, china. Scientific reports, 10(1), 6119.
Kiti, M. C., Tizzoni, M., Kinyanjui, T. M., Koech, D. C., Munywoki, P. K., Meriac, M., Cappa, L., Panisson, A., Barrat, A., Cattuto, C., et al. (2016). Quantifying social contacts in a household setting of rural kenya using wearable proximity sensors. EPJ data science, 5, 1–21.
Kiti, M. C., Melegaro, A., Cattuto, C., & Nokes, D. J. (2019). Study design and protocol for investigating social network patterns in rural and urban schools and households in a coastal setting in kenya using wearable proximity sensors. Wellcome open
research, 4.
Leecaster, M., Toth, D. J., Pettey, W. B., Rainey, J. J., Gao, H., Uzicanin, A., & Samore, M. (2016). Estimates of social contact in a middle school based on self-report and wireless sensor data. PloS one, 11(4), e0153690.
Machens, A., Gesualdo, F., Rizzo, C., Tozzi, A. E., Barrat, A., & Cattuto, C. (2013). An infectious disease model on empirical networks of human contact: Bridging the gap between dynamic network data and contact matrices. BMC infectious diseases, 13, 1–15.
Ozella, L., Paolotti, D., Lichand, G., Rodríguez, J. P., Haenni, S., Phuka, J., Leal-Neto, O. B., & Cattuto, C. (2021). Using wearable proximity sensors to characterize social contact patterns in a village of rural malawi. EPJ Data Science, 10(1), 46.5
Read, J., Edmunds, W., Riley, S., Lessler, J., & Cummings, D. (2012). Close encounters of the infectious kind: Methods to measure social mixing behaviour. Epidemiology & infection, 140(12), 2117–2130.
Smieszek, T., Barclay, V. C., Seeni, I., Rainey, J. J., Gao, H., Uzicanin, A., & Salathé, M.(2014). How should social mixing be measured: Comparing web-based survey and sensor-based methods. BMC infectious diseases, 14, 1–13.
Smieszek, T., Castell, S., Barrat, A., Cattuto, C., White, P. J., & Krause, G. (2016). Contact diaries versus wearable proximity sensors in measuring contact patterns at a conference: Method comparison and participants’ attitudes. BMC infectious diseases, 16, 1–14.